Chemical evolution of lithium
Machine learning to help understand a puzzling chemical element.
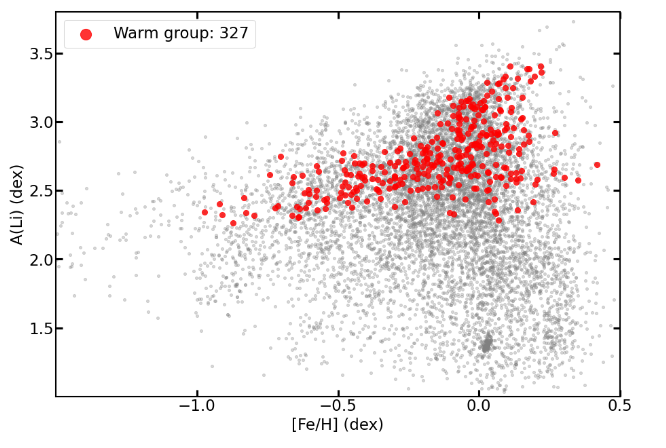
Lithium is a light element with atomic number 3 and two stable isotopes, with mass numbers 6 and 7. The 7Li isotope is the heaviest of the chemical elements, after the isotopes of H and He, which are produced in significant amounts in the so-called primordial nucleosynthesis that took place just a few minutes after the Big Bang. Despite its chemical simplicity, Li is one of the elements with the most complex behaviours in astrophysics. The behaviour of Li abundances in stars and in the Galaxy is one important open problem that astronomers are currently trying to solve.
In addition to the Big Bang, Li can be produced in several other astrophysical environments, including inside stars, by cosmic rays in the interstellar medium, and in nova explosions. In addition, it is a fragile element that can be quickly destroyed in reactions with protons when the temperature reaches about 2.5 million K or more, which is a relatively low temperature in the stellar interior. Due to its ease of production and destruction, Li exhibits behaviour that has so far eluded complete understanding.
To better understand Li in the context of both stellar and Galactic chemical evolution, it is necessary to determine Li abundances in a large number of stars covering a range of different properties, such as evolutionary stages, ages, metallicities, and Galactic volume. This was the goal of this work.
The analysis using machine learning
For the analysis, this work used a class of neural networks called convolutional neural networks (CNN). The network is trained to understand the variation of features associated with a set of reference spectra characterised by certain labels, the so-called “training sample”. The labels are the parameters and/or abundances of each star, including temperature, gravity, metallicity, and Li abundance. After the training stage, the same CNN can be used to infer the labels of another set of data. In this work, the CNN was trained on results of the Gaia-ESO survey and then used to infer the atmospheric parameters and lithium abundances for almost 40 000 stars. The codes and scripts used to build the CNN architecture are available online via a GitHub repository (see the column on the right).
The importance of the results
The CNN properly learnt the physics of the stellar labels and successfully derived the lithium abundances using the line at 6707.8 Å found in the Gaia-ESO GIRAFFE spectra. Rare objects such as lithium-rich giants were found. The figure shows the derived Li abundances as a function of metallicity. One can see the wide range of Li abundances covered by the stars, a result of Li depletion in their atmospheres. The group of "warm" stars marked in red have surface temperatures above 6800 K, and it is believed that they do not undergo Li depletion because they have thin convective layers. They constitute the best stars for studying the Galactic evolution of Li.
The results do not show the steep rise of the upper boundary of lithium abundances in the metallicity range between -1 < [Fe/H] < 0 dex, which is usually reported. Here, the stars seem to exhibit a shallower rise of the abundance, indicating early Li production by stars of high mass and a long delay in the production of Li by low-mass stars, in agreement with recent suggestions of theoretical models. We confirm that CNNs can be very useful in determining Li abundances in large data sets, such as the ones that will soon become available with the 4MOST and WEAVE large spectroscopic surveys.
This study was made by an international team of astronomers led by Samir Nepal, Ph.D. student at the Leibniz-Institut für Astrophysik Potsdam (AIP), Germany, with the collaboration of Dr. Maria Luiza Linhares Dantas, a postdoctoral researcher at the Nicolaus Copernicus Astronomical Centre (CAMK) and Rodolfo Smiljanic, associate professor at CAMK.
Text by Rodolfo Smiljanic